5 Effective Product Recommendation Strategies And How To Optimize Them In Today's Climate
By Pritwijit Mukherjee, Wipro Limited
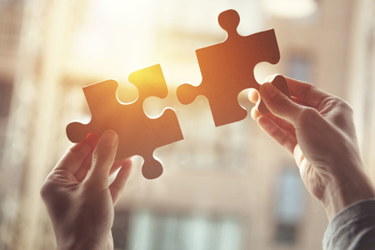
As we embrace a change in shopping behavior due to COVID-19, e-commerce marketers need to have smart product recommendation strategies to boost shopper conversion rates. According to NRF, 45% of shoppers are prioritizing need-oriented shopping over desire-oriented shopping – implying there is a need to understand new buying patterns. In this article, let’s explore five effective product recommendation strategies and three methods for optimizing customer experience with recommendations.
Type 1: Exploratory Recommendation Strategies
These are non-personalized product recommendation strategies that can reflect merchandise variety. These work well with impulsive personas looking to explore new products. This can generate merchandise awareness and capture traffic from top of the funnel. Suitable pages for placing these recommendations are the Home page, Category List Page, and Product List Page.
Method: Custom rules & descriptive analytics of historical sales can implement such strategies
Type 2: Cross-Sell
These are non-personalized product recommendation strategies that work well when shoppers are at the final stages of their buying journey – “consideration” or “purchase” stage. Page types suited for placing these recommendations are Product, Cart & Check-out pages
Method: These can be driven by merchandiser’s rules, and Machine Learning techniques such as Association Mining to suggest “associated products” that lift the odds of purchase when they are presented together to the shopper
Type 3: Similar Customer Based
This is the first step toward personalization as products are recommended based on behavioral patterns of similar shoppers. “Similar shoppers” can be identified through certain commonalities observed in browse and purchase patterns – for example similarity in products viewed, similarity in products purchased, similarity in product ratings, social media shares, etc.
Method: “People like you also like this” is based on a popular method “User based collaborative filtering” that recommends products based on preferences of similarly behaving shoppers. Since there is no restriction on product category, this recommendation can present variety to the shopper, and hence suits better at a Home Page or Category Listing Page (CLP). Other recommendation strategies in this group are “People who viewed this, also viewed that”, which are based on the specific product viewed by the shopper.
Type 4: Up-Sell Or Similar Item Based
These recommendation strategies may or may not be personalized depending on the example. The 1st two examples - “Similar Products” and “More like this” result in products similar to the product being viewed. Such strategies result in the same product for every user. These work well for personas in “consideration” stage and looking for a specific product.
On the other hand, “Items similar to what you like” are personalized to the user’s historical preferences. This strategy is effective in re-engaging with existing customers when placed on Home, CLP, or Product pages.
Method: “Items similar to what you like” are driven by item-based collaborative filtering based on user history. “More Like This” & “Similar Products” can be driven by item-based collaborative filtering or even by custom rule-based similarities, such as common product attributes, common audience sentiments, etc.
Type 5: Feature Affinity Based
These are the most personalized recommendations since they are based on feature affinities of individual shoppers. These can be used for personas in “awareness” stage as they can be made aware of new products having features they prefer.
Method: These recommendations can be driven by Matrix Factorization, which uses historical data to implicitly arrive at abstract features responsible for driving positive shopper behavior. Since these features are implicitly derived by machines, there is a possibility that products scoring high for these features appear across different categories. For example, a feature could represent “Fashion Forward” and products scoring high in this attribute could be across categories – such as fashion-forward jeans, fashion-forward sweatshirts, etc. Suitable pages are Home Page, CLP & Product Listing Page (PLP).
Optimizing Product Recommendations
Ecommerce marketers usually find competing recommendation strategies such as “New Arrivals” vs “Best Sellers” at a Home Page, “More Like This” vs “Viewed this, viewed that” on a Product Page. Optimizing product recommendations allows marketers to execute the best-performing recommendation strategy. Three methods for optimizing experiences with product recommendations are described below.
A/B Testing – Whenever there are competing product recommendation strategies, A/B testing can be used to find out the best strategy.
When to use this: This can work well when campaign goals allow a one-size-fits-all strategy and campaign duration allows sufficient time to collect data and learn.
Dynamic Optimization using Bandits: This method uses Reinforcement Learning to adapt product recommendation strategies based on responses received by visitors in real time. Let’s consider two variant strategies for Home Page – “Best Sellers” vs “New Arrivals”. AI initiates a random serving of a variant to the visitor - let’s say “Best Sellers”. It treats a visitor conversion as a reward and increments the score of “Best Sellers”. For the next visitor, AI chooses a variant based on the updated scores, i.e. its learning from the previous responses.
When to use this: This works best for short seasonal campaigns, for example, CyberMonday, when marketers want a tactical one-size-fits-all strategy for all visitors at the top of their Home Page and need to choose from competing options – such as “New arrivals” and “Bestselling items this season” but cannot afford the time to run A/B test experiments.
Dynamic Personalization Through Contextual Bandits
This method is used to personalize experiences by incorporating visitor context, unlike the previous methods. It is used to predict the best product recommendation strategy for each visitor based on their context features in real time – such as demographics, technographics, session behavior, historical purchases, location & weather, etc.
When to use this: Marketers can use this when they want to deliver 1:1 experiences based on shopper context. For example, on a Product page, should we prioritize “Goes Well With” i.e. cross-selling or should we prioritize “More Like This” or similar products? Some personas will be need-oriented and will engage more with similar products, while impulsive personas looking for discovery may engage more with accessories
Where To Start?
The right personalization roadmap for retailers depends on their maturity in data & intelligence. To effectively embark on a personalization journey, retailers need to combine domain and technical expertise, and also leverage commercial platforms available in the market. As consumers continue to shop online, let’s re-assess our maturities and have the right roadmap for dynamic personalization.