Looking To Bounce Back After COVID-19? How AI & Data Analytics Can Help
By Pedro Alves, Ople.ai
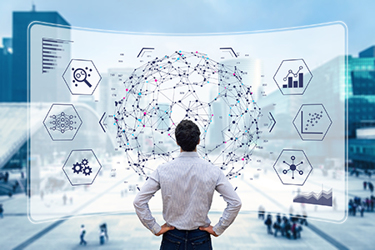
Up until recently, most efforts with AI in retail fell under the umbrella of “optimization.” For the online shopping experience, AI-powered tools provide retailers with product recommendations to show customers to increase sales, or the system offers a different UI for different customers. These are all perfectly fine usages that can boost sales and profits, but they are not doing much on the customer experience side, especially with the “new normal” for retail and e-commerce.
With retail moving to predominately online models, there are more opportunities than ever for AI to shine. Not just for further optimization, but to create new shopping experiences. This means finding ways to bring the best parts of in-person shopping seamlessly into the advantages of online shopping such as instantaneous ordering, the breadth of products, and the ability to access supporting information such as product descriptions and reviews.
Getting into AI
A first step before diving headfirst into AI is to speak with someone in the industry. This could be an advisor or consultant, or a meeting with an AI software provider who understands the retail space. The point of an initial meeting with an AI advisor is to take stock of where the retailer is now and to determine what they need. They might tell the retailer they aren’t ready for AI, and first need to improve their data collection or management practices.
AI can impact retailers on multiple fronts, from inventory and logistics to site optimization. That is its power, but also possibly a limitation because it means users can run the risk of losing focus. To avoid this, retailers need to narrow down their business challenges and see what types of related data they have on hand. They can then judge whether the challenges warrant hiring staff or if they can rely on AI software.
Platforms such as Ople.ai offer retailers accessible and impactful machine learning capabilities that can power various tools and inform business decisions. Retailers can leverage more out of such tools when the insights it produces are usable by the logistics manager, the marketing crew, and the website design team. Each group plays an important role for retailers to remove friction and increase relevancy. Together, they can use AI to go beyond optimization and create a new type of post-COVID shopping experience.
Retail Use Cases
For apparel, some retailers have utilized computer vision tools enhanced with AI to allow customers to “try on” clothing to gauge the fit. For retailers, a streamlined tool that helps shoppers check sizing and fit can drastically reduce cumbersome returns which costs the retailers money and dampen the shopping experience. Beyond sizing, additional tools use augmented reality and AI to enable shoppers to “see” an item on their own body. Some companies currently use this type of technology, but it is often inexact. They might ask the shopper to offer their “body type” data, and then present a virtual model that is similar in size. AI can improve these tools by allowing the individual shopper to become the model.
Retailer Neiman Marcus offers an app feature called Snap.Find.Shop., which uses AI and computer vision to enable people to take an image of an item such as a pair of shoes or scarf, which then finds similar items that are available on the website. It’s an evolution of the common “customers also bought” feature, where the system takes advanced physical elements from an item and presents a relevant purchasing alternative.
On the back end, AI can help retailers locate areas for cost reductions. In a retail environment where the volume for every item is depressed, retailers need intelligent guidance on where to spend their money and what to stock. They can use AI to streamline stock and logistics processes to cut waste, eliminate “out of stock” problems, and improve inventory replenishment.
About The Author
Pedro Alves is the founder and CEO of Ople.AI, a software startup that provides an Automated Machine Learning platform to empower business users with predictive analytics. While pursuing his Ph.D. in Computational Biology from Yale University, Alves started his career as a data scientist and gained experience in predicting, analyzing, and visualizing data in the fields of social graphs, genomics, gene networks, cancer metastasis, insurance fraud, soccer strategies, joint injuries, human attraction, spam detection, and topic modeling among others. Realizing that he was learning by observing how algorithms learn from processing different models, Alves discovered that data scientists could benefit from AI that mimics this behavior of learning to learn. Therefore, he founded Ople to advance the field of data science and make AI easy, cheap, and ubiquitous.