Using Predictive Analytics To Help Solve Retailers' Challenges

By Christine Kern, contributing writer
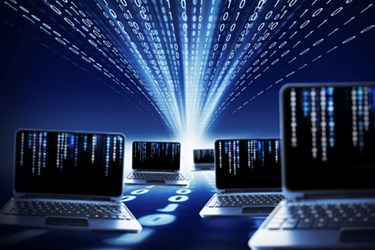
How to solve the most common retail data problems to improve efficiency and boost sales.
Big data is poised in the coming years to open up huge opportunities in the way both physical and online retail stores fundamentally operate and serve customers. All retail companies face 4 fundamental challenges that can be solved with predictive analytics and an effective data production plan, including:
- Siloed, Static Customer Views
- Time Consuming Vendor & Supply Chain Management
- Analysis Based on Historical Data
- One-Time Data Projects
To help retailers leverage predictive analytics to address these problems, data science software maker, Dataiku, has published a free guide that outlines the problems and solutions facing retailers today. The most successful retail companies are utilizing data science and predictive analytics to improve efficiency, improve marketing campaigns, and gain significant customer insight for a competitive advantage.
In 4 Big Challenges for Retailers, Solved with Predictive Analytics, data science software maker, Dataiku, recently explored the types of data problems facing retail, the problems they solve, and the steps that any retail organization can take to become more data driven.
PROBLEM #1: Siloed, Static Customer Views
To counter the problem faced by many retailers of siloed data, in which transaction data lives apart from web logs which in turn is separate from CRM data, retailers can turn to complete, real-time customer looks. Cutting-edge retailers look at customers as a whole, combining traditional data sources with the non-traditional like social media or other external data sources to create valuable insight, resulting in robust fraud detection systems, more effective marketing campaigns, more accurate and targeted churn prediction, and better customer service.
PROBLEM #2: Time Consuming Vendor & Supply Chain Management
While supply chains are already being driven by numbers and analytics, retailers have been slower to embrace the power of real-time analytics and harnessing huge, unstructured data sets. With automation and prediction for faster, more accurate management, retailers can combined structured and unstructured data in real time to create more accurate forecasts or automatic reordering, resulting in optimized pricing strategies and more efficient inventory management.
PROBLEM #3: Analysis Based on Historical Data
While a shopper’s past activity can be interesting, it often isn’t a good indication of what they will do next. Instead, retailers need to use prediction and machine learning in real time to create predictions based on current behaviors and trends. This allows retailers to anticipate a customer’s next move, while creating a more agile business based on up-to-the-minute signals with the ability to adapt automatically to customer behaviors.
PROBLEM #4: One-Time Data Projects
Retailers are often frustrated by one-off data projects that aren’t reproducible. To create more efficient results, retailers should turn to automated, scalable, and reproducible data initiatives that allow retail data teams to focus on implementing an efficient project that can be replicated. By creating reproducible workflows, the team can more on more projects, improving overall efficiency in the process.